Can AI accurately detect harmful content?
The most effective measure in preventing the January 6 Capitol insurrection may have not been President Donald Trump or the FBI but computer science. Researchers discovered that many rioters planned the attack on social media months preceding the protests. While critics blame social media platforms for failing to crack down on extremist groups, law enforcement officials, federal agencies, and social media companies did not detect posts, messages, and tweets that indicated political violence. To track violent language on social media, tech companies and researchers are developing artificial intelligence (AI) to detect hate-speech online before it materializes into violence in the real world.
Users reporting harmful content is the simplest way to tackle hate on social media platforms. However, hateful incidents—–including hate crimes and speech—are significantly underreported. Human detection heavily depends on individual bias and subjectivity. Additionally, hate speech can also be difficult to classify due to time. There are millions of hate speech cases reported on social media platforms each year. As a result, social media companies do not have the time to carefully analyze each report.
Instead, scientists have turned to AI. To automatically detect hateful posts, social media companies use this algorithm-based technology to identify hate speech. Artificial intelligence techniques used in this context include Term Frequency-Inverse Document Frequency (TF-IDF), Point-Wise Mutual Information (PMI), and logistic regressions.
TF-IDF quantifies the significance of a word in a text by assigning each word a numerical value. For example, in the case of hate speech, the computer analyzes a group of tweets, and the text includes the sentence “I hate people.” While humans can understand this sentence because they know the significance of each word, the computer can only understand the text in terms of numerical values. The TF-IDF assigns numerical scores to a term based on the frequency that the term appears in the text. To track hate-speech, data sets assign TDF-IDF scores to words based on how relevant that word is in a dataset of text on social media platforms.
The PMI technique calculates the correlation of a word in a text to certain categories
such as ethnicity, religion, and disability. For instance, researchers would calculate the correlation of words like “ape” or “filthy” to categories, such as ethnicity and social class, often used to denigrate individuals. Computer scientists aim to accurately correlate words to two categories: hate or non-hate speech. Research from an HSLT development group in Spain shows that a PMI algorithm classifies the most derogatory ethnic terms as highly correlated to hateful speech while the least hateful words (i.e. happy, idea, french) as having a significantly low correlation.
Mathematicians use logistic regressions to predict the result of a binary outcome, a situation in which only two possible scenarios can occur. If the event happens, the probability is 1, and if it does not happen, the probability is 0. The logistic model applies to text in a dataset to a binary dependent variable. To detect hate speech, researchers use a logistic model to determine the probability that a text is hate speech. The final value of the dependent variable is hate speech (1) and non-hate speech (0). The probability of the dependent variable depends on the independent variables, which are text-related parameters assigned to the content in a text such as TDF-IDF scores.
While these traditional models have shown reasonable accuracy––especially the logistic model at around 70 percent––in detecting hate speech, these models have exhibited biases and prejudices. For instance, the University of Washington and Carnegie Mellon University researchers found that artificial intelligence and machine learning models are 1.5 times more likely to label tweets by African-Americans as offensive, especially if these tweets were in African American Vernacular English. Although artificial intelligence models generally identify keywords frequently used in hate speech, they do not accurately evaluate the context in which the words are used. For example, hate-speech classifiers are often oversensitive to group identifiers like “black,” “Muslim,” and “women” because these terms often appear in a hateful. Even if researchers use the TFD-IDF numerical values to computationally signify terms, the computer is still limited in understanding text holistically.
To combat this issue, a team of University of South California researchers developed a context-sensitive hate-speech classifier, which uses a technique that trains an algorithm to analyze the context in which group identifiers are used and whether features—such as insulting language—of hate speech are present. For example, if a computer is classifying the phrase “said one Muslim shop owner,” instead of grouping “one Muslim shop owner” as hate speech, it considers the word “said” to identify the phrase as attribution to a quote.
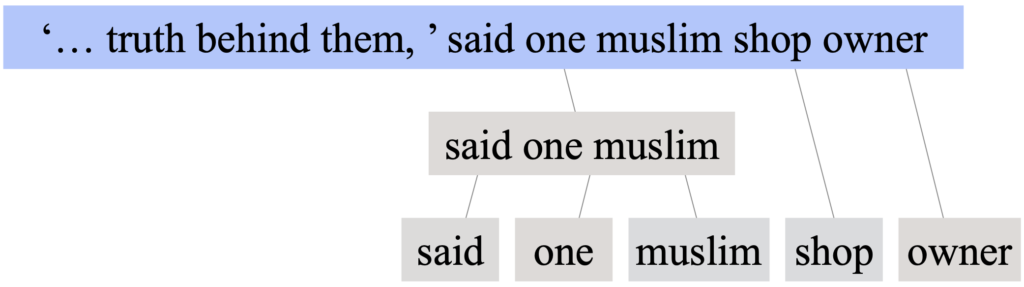
The USC model aims to help fix this problem by using more balanced datasets. Algorithms that identify hate speech are generally based on datasets with a high rate of hate speech, such as white supremacists’ forums. Therefore, these imbalanced datasets can often lead to algorithms discriminating against the use of group identifiers. Professor Xiang Ren, one of the researchers in the USC study, noted that “if you teach a model from an imbalanced dataset, the model starts picking up weird patterns and blocking users inappropriately.” By using more comprehensive datasets, the USC model has shown promising results. As compared to traditional models in differentiating hate and non-hate speech in New York Times articles, it achieved 90% percent accuracy compared to a typical 77% accuracy.
While increasing the data used to program algorithms may make automatic detection more viable, it is not necessarily sustainable. For example, Facebook implemented this method; however, the company still has not effectively cut hate speech on its platform. Although Facebook claims that it now accurately detects 94.7 percent of removed hate speech, harmful content still spreads on the site. Since data rapidly evolves, additional, but out-of-context, data does not necessarily manifest in increased accuracy of hate-speech identifiers.
Still, hate speech does not have a universal definition, and humans continually evolve their idea of what it is. If humans cannot define hate speech, how can computers? While AI has proven to be a powerful tool in this fight, computers cannot critically think in novel situations like humans. Likely, the future of hate speech prevention will include a hybrid of human and artificial intelligence.
– Gerson Personnat
Image Sources